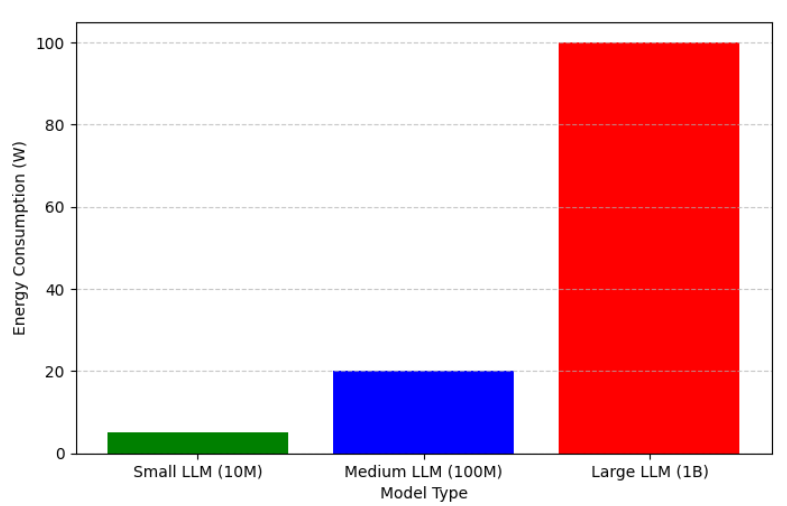
Downloads
Keywords:
EdgeAI for Privacy-Preserving AI: The Role of Small LLMs in Federated Learning Environments
Authors
Abstract
Privacy considerations in artificial intelligence (AI) have led to the popularization of federated learning (FL) as a decentralized training organization. On this basis, FL allows collaborative model training without requiring data exchange for private data use. The adoption of FL on edge devices faces major challenges due to limited computational resources, networks, and energy efficiency. This paper analyzes the operation of small language models (SLMs) in FL frameworks with an eye on their promise to let intelligent privacy-preserving architectures thrive on edge devices. It is through SLMs that local inference can be made robust while exposing less data. This research investigates the performance of SLMs under different TinyML applications such as natural language understanding and anomaly detection, along with the inherent security vulnerabilities of SLMs in federated learning environments compared to other attack scenarios. Furthermore, effective countermeasures are proposed. Only the policy implications of adopting SLMs for privacy-sensitive domains will be covered, advocating for governance policy frameworks that delicately balance innovations and data protection.
Article Details
Published
Issue
Section
License

This work is licensed under a Creative Commons Attribution-NonCommercial-ShareAlike 4.0 International License.