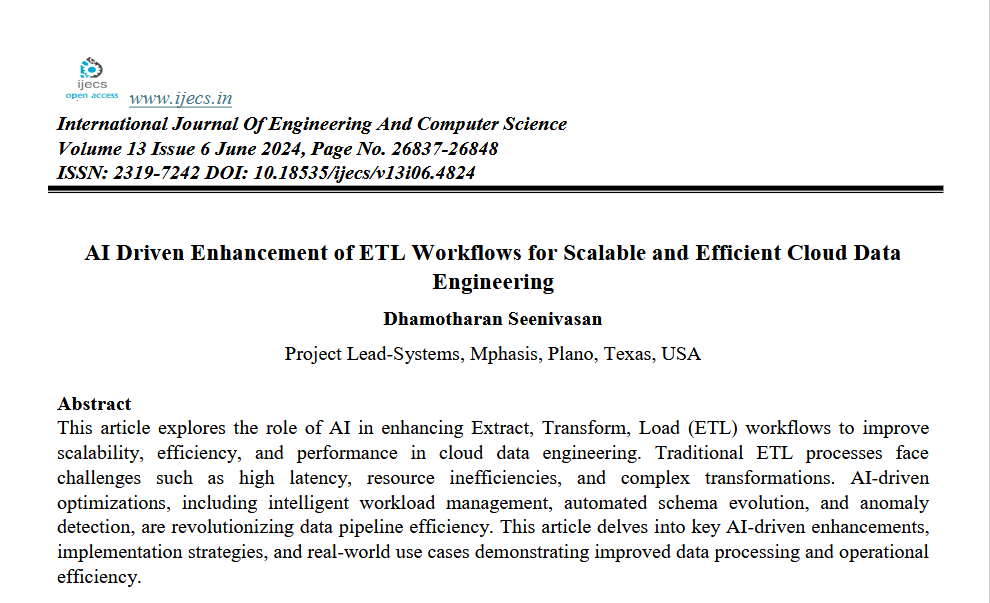
Downloads
Keywords:
AI-driven ETL; Cloud data engineering; ETL workflows; Machine learning in ETL; Real-time ETL processing; No-code ETL platforms; Low-code AI platforms; Cloud-based ETL tools
AI Driven Enhancement of ETL Workflows for Scalable and Efficient Cloud Data Engineering
Authors
Abstract
This article explores the role of AI in enhancing Extract, Transform, Load (ETL) workflows to improve scalability, efficiency, and performance in cloud data engineering. Traditional ETL processes face challenges such as high latency, resource inefficiencies, and complex transformations. AI-driven optimizations, including intelligent workload management, automated schema evolution, and anomaly detection, are revolutionizing data pipeline efficiency. This article delves into key AI-driven enhancements, implementation strategies, and real-world use cases demonstrating improved data processing and operational efficiency.
Article Details
Published
2024-06-28
Issue
Section
Articles
License
Copyright (c) 2024 International Journal of Engineering and Computer Science


This work is licensed under a Creative Commons Attribution-NonCommercial-ShareAlike 4.0 International License.
How to Cite
AI Driven Enhancement of ETL Workflows for Scalable and Efficient Cloud Data Engineering. (2024). International Journal of Engineering and Computer Science, 13(06), 26837-26848. https://doi.org/10.18535/ijecs.v13i06.4824