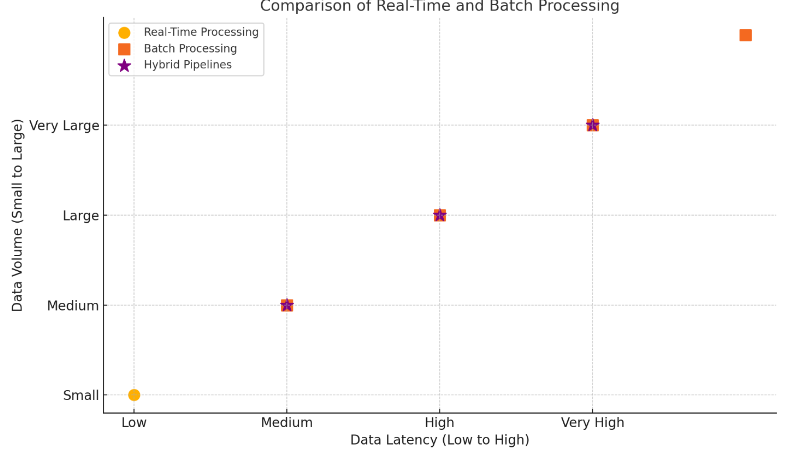
Downloads
Keywords:
Scalable AI Pipelines in Edge-Cloud Environments: Challenges and Solutions for Big Data Processing
Authors
Abstract
The increasing need for Scable and efficient data processing has seen the integration of Edge computing and cloud computin, which presents a sound architecture for deployment of AI pipelines. These are the ‘hybrid’ environments to capture the massive and highly varied data being delivered in today’s solutions across sectors like healthcare, smart cities and industrial IoT. Yet, AI pipeline construction in edge-cloud settings raises several issues, mainly resources’ heterogeneity, latency and bandwidth constraints, security, cost.
This paper aims at discussing the following challenges and afterward proposing a solution that embraces the help of emerging technologies. Apache Spark is the kind of distributed processing that makes handling large data possible; on the other hand, federated learning allows decentralized AI model training and avoids extensive data transfer. Microservices architectures and containerization, through Docker, and Kubernetes make modularity and scalability easier. Additionally, the research considers adaptive resource management approaches and edge-cloud cooperation patterns to understand the workload distribution optimisation.
In this study, the systematic literature review and analysis of current ongoing applications help to determine best practices and trends defining the direction for the future scalable AI pipeline. It also explains how existing limitations are addressed by the enabling technologies that include 5G, block chain and AI- based orchestration. Last, this paper emphasizes on the need for strengthening uniformity, cooperation between the organizations and constancy in the enhancement of edges–cloud ecosystems for big data analytics. In addressing these considerations, this work will offer a roadmap for researchers and practitioners who want to build robust and elastic AI systems to succeed in the data-intensive world.
Article Details
Published
Issue
Section
License

This work is licensed under a Creative Commons Attribution-NonCommercial-ShareAlike 4.0 International License.